game | pitch | twogoals | nplayer | ntouches | eps |
---|---|---|---|---|---|
G1 | large | yes | 6p | free | 68.5 |
G1 | large | no | 5p | free | 66.8 |
G1 | large | yes | 5p | restricted | 58.5 |
G1 | large | no | 6p | free | 70.8 |
G1 | large | yes | 6p | restricted | 61.3 |
G1 | large | no | 5p | restricted | 51.9 |
27 Split-plot Design
27.1 Farming
27.2 CRD
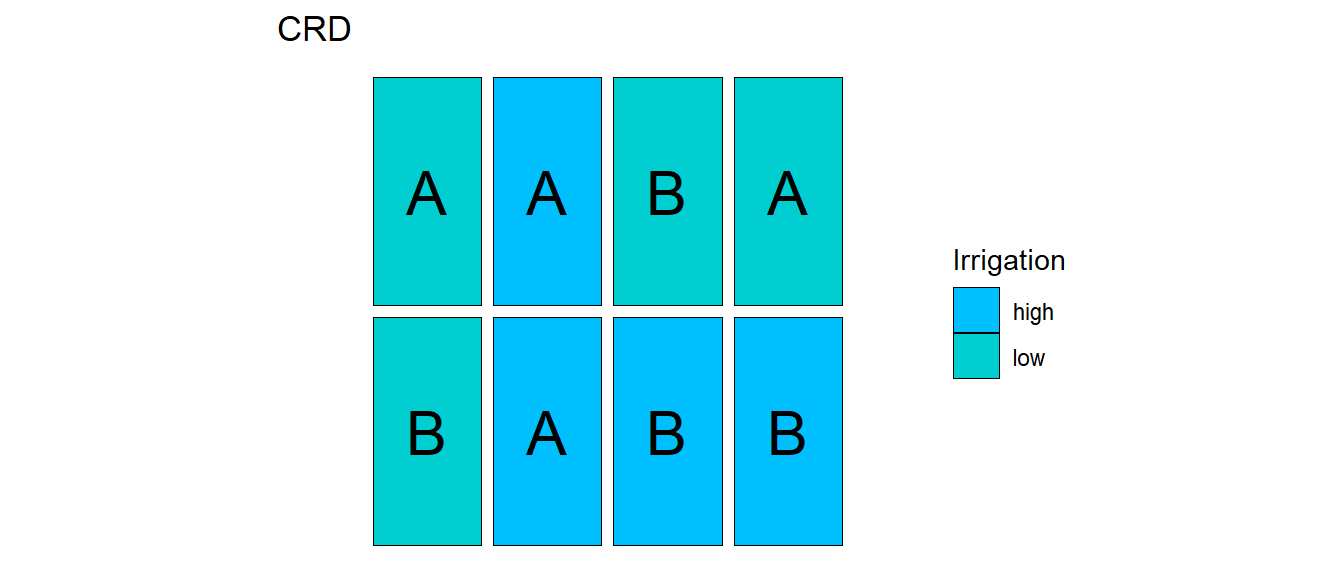
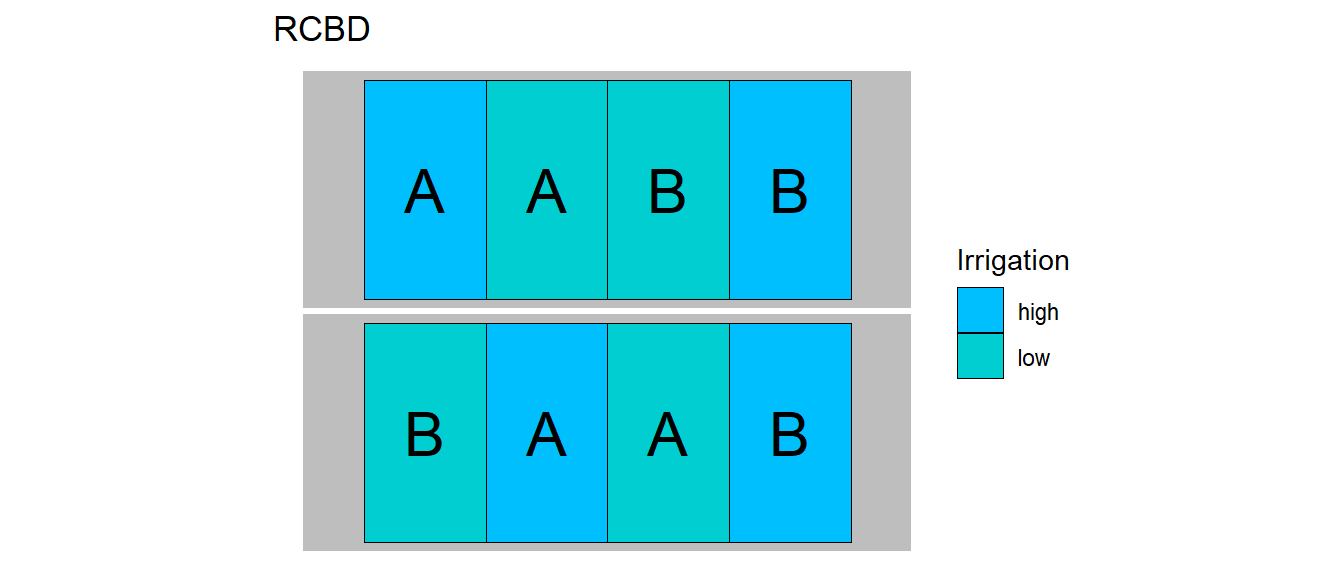
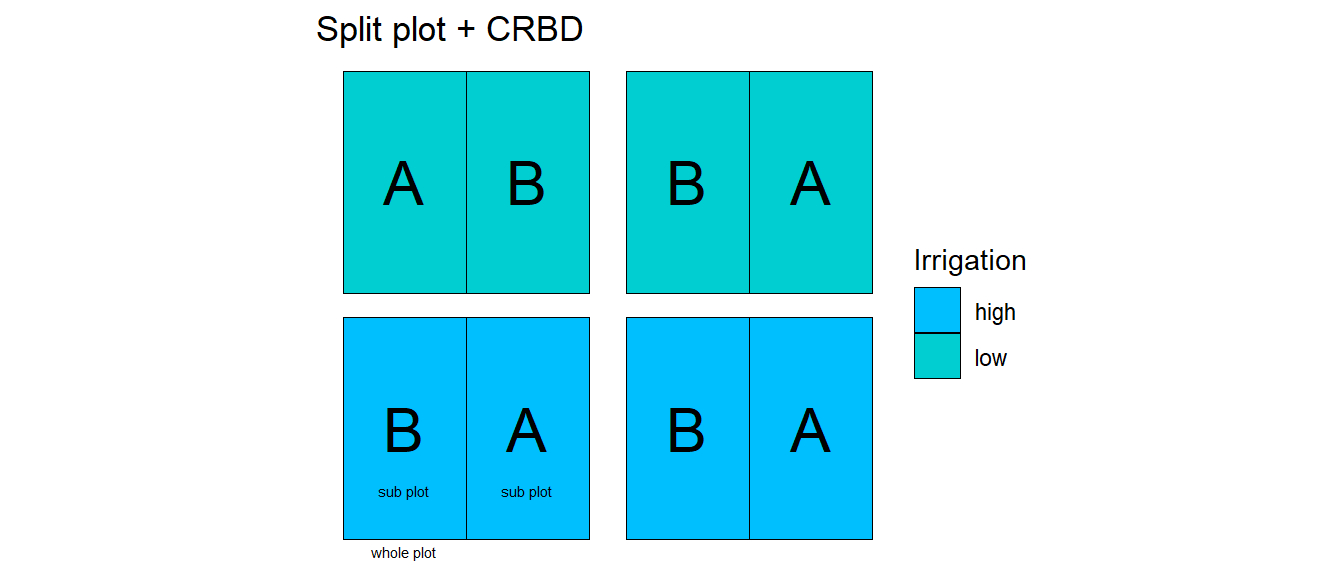
27.3 Example - Soccer
Das folgende hypothetische Beispiel ist adaptiert nach Kowalski et al. (2007)]
27.4 Example - Soccer
27.5 Whole-plot analysis
27.6 Whole-plot analysis Analysis of variance
game | eps | pitch |
---|---|---|
G1 | 62.94 | large |
G2 | 57.81 | small |
G3 | 62.92 | small |
G4 | 64.34 | large |
<- aov(eps ~ pitch, df_bars)
mod_whole_plot summary(mod_whole_plot)
Df Sum Sq Mean Sq F value Pr(>F)
pitch 1 10.69 10.685 1.521 0.343
Residuals 2 14.05 7.024
27.7 Error components split-plot analysis
27.8 Complete Split-plot analysis
<- aov(eps ~ (pitch+nplayer+twogoals+ntouches)^2 + Error(game), df)
mod_sp summary(mod_sp)
Error: game
Df Sum Sq Mean Sq F value Pr(>F)
pitch 1 85.48 85.48 1.521 0.343
Residuals 2 112.39 56.20
Error: Within
Df Sum Sq Mean Sq F value Pr(>F)
nplayer 1 41.18 41.18 4.210 0.0542 .
twogoals 1 45.36 45.36 4.637 0.0443 *
ntouches 1 75.95 75.95 7.765 0.0118 *
pitch:nplayer 1 78.44 78.44 8.019 0.0107 *
pitch:twogoals 1 1.09 1.09 0.111 0.7424
pitch:ntouches 1 62.44 62.44 6.383 0.0206 *
nplayer:twogoals 1 27.94 27.94 2.856 0.1074
nplayer:ntouches 1 43.95 43.95 4.492 0.0474 *
twogoals:ntouches 1 2.94 2.94 0.301 0.5899
Residuals 19 185.86 9.78
---
Signif. codes: 0 '***' 0.001 '**' 0.01 '*' 0.05 '.' 0.1 ' ' 1
27.9 Model
\[\begin{align*} Y_{hij} &= \mu + \alpha_i + \epsilon_{i(h)}^W \\ & + \beta_j + (\alpha\beta)_{ij} + \epsilon_{j(hi)}^S \end{align*}\]
\(\epsilon_{jt(hi)}^S \sim \mathcal{N}(0,\sigma_s^2)\)
\(h=1,\ldots,s\)
\(i=1,\ldots,a\)
\(j=1,\ldots,b\)
27.10 A more common example
27.11 Data structure
id | time | group | CMJ |
---|---|---|---|
S1 | Pre | CON | 20.5 |
S2 | Pre | CON | 20.8 |
S11 | Pre | TRT | 20.9 |
S1 | Post | CON | 21.5 |
S11 | Post | TRT | 24.6 |
S3 | Ret | CON | 20.0 |
S14 | Ret | TRT | 22.4 |
27.12 Model
\[\begin{align*} Y_{hij} &= \mu + \theta_h + \alpha_i + \epsilon_{i(h)}^W \\ & + \beta_j + (\alpha\beta)_{ij} + \epsilon_{j(hi)}^S \end{align*}\]
\(\epsilon_{jt(hi)}^S \sim \mathcal{N}(0,\sigma_s^2)\)
\(h=1,\ldots,s\)
\(i=1,\ldots,a\)
\(j=1,\ldots,b\)
27.13 Split-plot analysis
<- aov(CMJ ~ group*time + Error(id), df_1)
mod summary(mod)
Error: id
Df Sum Sq Mean Sq F value Pr(>F)
group 1 70.01 70.01 94.31 1.4e-08 ***
Residuals 18 13.36 0.74
---
Signif. codes: 0 '***' 0.001 '**' 0.01 '*' 0.05 '.' 0.1 ' ' 1
Error: Within
Df Sum Sq Mean Sq F value Pr(>F)
time 2 98.87 49.43 44.58 1.82e-10 ***
group:time 2 42.94 21.47 19.36 1.95e-06 ***
Residuals 36 39.92 1.11
---
Signif. codes: 0 '***' 0.001 '**' 0.01 '*' 0.05 '.' 0.1 ' ' 1
27.14 Again, standard analysis is not correct!
<- aov(CMJ ~ id + group*time, df_1)
mod_falsch summary(mod_falsch)
Df Sum Sq Mean Sq F value Pr(>F)
id 19 83.38 4.39 3.957 0.000189 ***
time 2 98.87 49.43 44.579 1.82e-10 ***
group:time 2 42.94 21.47 19.363 1.95e-06 ***
Residuals 36 39.92 1.11
---
Signif. codes: 0 '***' 0.001 '**' 0.01 '*' 0.05 '.' 0.1 ' ' 1
27.15 Alternative analysis using mixed models
<- lmer(CMJ ~ time*group + (1|id), df_1)
mod_lmer anova(mod_lmer)
Analysis of Variance Table
npar Sum Sq Mean Sq F value
time 2 98.866 49.433 50.099
group 1 70.013 70.013 70.957
time:group 2 42.944 21.472 21.761
27.16 Effect size
\[\begin{align*} \hat{\omega}^2_{\text{between}} &= \frac{SS_A -(a-1)MS_{S/A}}{SS_A + SS_{S/A}+MS_{S/A}} \\ \hat{\omega}^2_{\text{within}} &= \frac{(b-1)(MS_b - MS_{B\times S/A})}{SS_B + SS_{B\times S/A}+SS_{S/A}+MS_{S/A}} \\ \hat{\omega}^2_{AB} &= \frac{(a-1)(b-1)(MS_{AB}-MS_{B\times S/A})}{SS_{AB}+SS_{B\times S/A}+MS_{S/A}} \end{align*}\]
group
, \(B=\)time
, \(AB=\)group:time
, \(S/B=\)Error: id
, \(B\times S/A=\)Error: within
im Beispiel27.17 Effect size in R
::omega_squared(mod) effectsize
# Effect Size for ANOVA (Type I)
Group | Parameter | Omega2 (partial) | 95% CI
-----------------------------------------------------
id | group | 0.82 | [0.67, 1.00]
Within | time | 0.63 | [0.46, 1.00]
Within | group:time | 0.42 | [0.20, 1.00]
- One-sided CIs: upper bound fixed at [1.00].
27.18 Multiple comparisons
<- emmeans(mod, ~time*group)
mod_em pairs(mod_em)
contrast estimate SE df t.ratio p.value
Pre CON - Post CON -1.271 0.471 36.0 -2.699 0.1002
Pre CON - Ret CON 0.340 0.471 36.0 0.722 0.9780
Pre CON - Pre TRT 0.226 0.444 52.4 0.509 0.9956
Pre CON - Post TRT -4.775 0.444 52.4 -10.750 <.0001
Pre CON - Ret TRT -2.863 0.444 52.4 -6.445 <.0001
Post CON - Ret CON 1.611 0.471 36.0 3.421 0.0181
Post CON - Pre TRT 1.497 0.444 52.4 3.370 0.0168
Post CON - Post TRT -3.504 0.444 52.4 -7.888 <.0001
Post CON - Ret TRT -1.592 0.444 52.4 -3.584 0.0092
Ret CON - Pre TRT -0.114 0.444 52.4 -0.256 0.9998
Ret CON - Post TRT -5.115 0.444 52.4 -11.515 <.0001
Ret CON - Ret TRT -3.203 0.444 52.4 -7.211 <.0001
Pre TRT - Post TRT -5.002 0.471 36.0 -10.621 <.0001
Pre TRT - Ret TRT -3.089 0.471 36.0 -6.560 <.0001
Post TRT - Ret TRT 1.912 0.471 36.0 4.060 0.0032
P value adjustment: tukey method for comparing a family of 6 estimates
27.19 Which comparisons are meaninful?!
<- emmeans(mod, ~time|group)
mod_em2 pairs(mod_em2)
group = CON:
contrast estimate SE df t.ratio p.value
Pre - Post -1.27 0.471 36 -2.699 0.0277
Pre - Ret 0.34 0.471 36 0.722 0.7523
Post - Ret 1.61 0.471 36 3.421 0.0044
group = TRT:
contrast estimate SE df t.ratio p.value
Pre - Post -5.00 0.471 36 -10.621 <.0001
Pre - Ret -3.09 0.471 36 -6.560 <.0001
Post - Ret 1.91 0.471 36 4.060 0.0007
P value adjustment: tukey method for comparing a family of 3 estimates
27.20 Further reading
27.20.1 Allgemein
Kutner u. a. (2005, p.1172), Kowalski, Parker, und Geoffrey Vining (2007), Altman und Krzywinski (2015)